Artificial intelligence: trustworthiness of neural networks
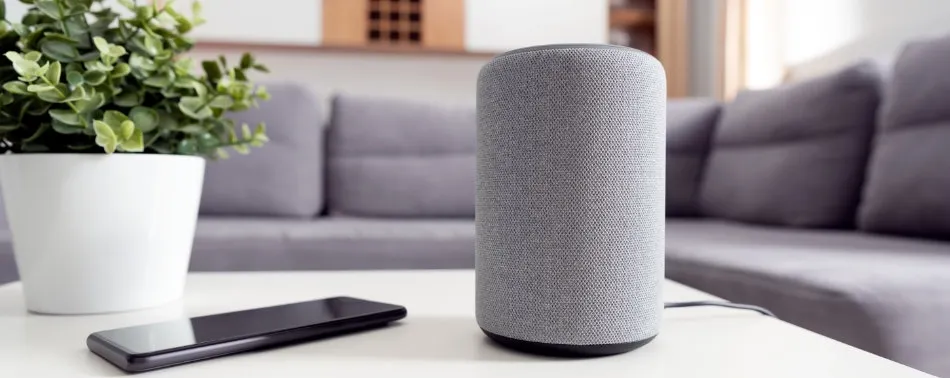
A neural network is a type of artificial intelligence (AI) inspired by the structure and functioning of the human brain. Neural network technology is found in a wide range of applications that are shaping the way we interact with the world, from voice assistants like Alexa or Siri to self-driving vehicles and personalized recommendation systems.
Neural networks consist of interconnected nodes, also known as artificial neurons or units, organized in layers. Each neuron takes input, processes it using a specific weight and bias and produces an output that is fed forward to the next layer.
Neural networks learn from data through a process called training, where they adjust their weights and biases to make better predictions or decisions over time. They have become an essential part of modern AI systems due to their ability to process unstructured data (like images or sounds), identify patterns and make predictions.
They have achieved remarkable success in various applications, including computer vision, natural language processing, speech recognition and more. As AI technology integrates deeper into our lives, however, there is a growing concern for its trustworthiness. This concern comes from two factors intrinsic to neural networks: the difficulty to explain their output and the difficulty to predict their behaviour everywhere in their domain of use.
Can we rely on AI systems to make accurate decisions, especially when unforeseen circumstances arise? In critical areas such as healthcare, finance and self-driving vehicles, AI errors can have an important impact on the trustworthiness of the system and in return its acceptability by the public or the industry. ISO/IEC 24029 series help to address these concerns.
Robustness is the ability of an AI system to maintain its level of performance under any conditions. The technical report, ISO/IEC TR 24029-1, published in 2021, highlights three types of methods that can be used to assess the robustness of neural networks:
- Formal methods rely on sound formal proofs to check if certain properties are provable over a specific domain of use. For example, evaluators can assess whether the system always operates within specified safety boundaries;
- Statistical approaches involve mathematical testing on datasets to determine a certain level of confidence in the results. They help evaluators answer questions related to performance thresholds, such as false positive/negative rates, and whether they are acceptable;
- Empirical methods: These methods involve experimentation, observation, and expert judgment to assess the system's behaviour in specific scenarios. Evaluators can determine the degree to which the system's properties hold true in real-life situations.
The newly published international standard, ISO/IEC 24029-2 focuses on formal assessment methods for gauging the robustness of neural networks.
All these standards and documents can be found in our e-shop.